ML Model - ML Model Analysis Tool
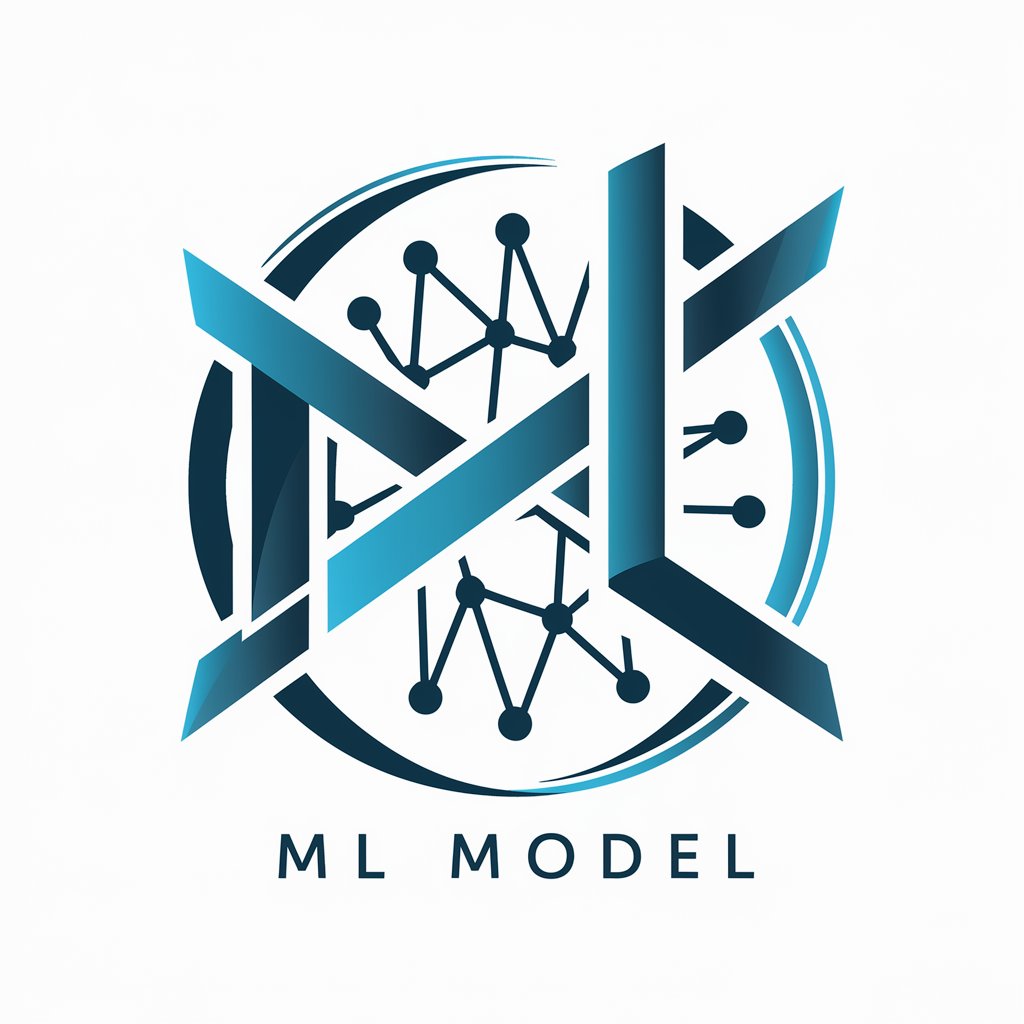
Welcome to ML Model, your guide to precise machine learning analysis.
Optimize ML performance with AI-driven insights
Compare the top three machine learning models for...
Analyze the impact of feature selection on...
Determine the highest accuracy model for...
Evaluate the performance of different models with and without...
Get Embed Code
Overview of ML Model
ML Model is a specialized tool designed to offer comprehensive comparative analyses of various machine learning models, focusing on their performance in relation to feature selection. The core purpose of ML Model is to enable users to understand how the inclusion or exclusion of certain features within a dataset affects the accuracy and efficiency of different machine learning models. Through a detailed examination of datasets in two states—where non-important features are included and where they are excluded—ML Model provides insights into the impact of these features on model performance. This includes calculating and presenting the accuracy of each model under both scenarios, thereby assisting users in making informed decisions about feature importance and model selection. For example, if a user has a dataset for predicting housing prices, ML Model can analyze how different models perform with and without features like the house's age or proximity to amenities, offering a clear view of which features significantly influence predictions. Powered by ChatGPT-4o。
Key Functions of ML Model
Comparative Analysis of Machine Learning Models
Example
Comparing models like Random Forest, Gradient Boosting, and Neural Networks on a dataset for credit risk assessment.
Scenario
A financial institution wants to improve its loan approval process by identifying the most accurate machine learning model for predicting default risk. ML Model evaluates the models' performance with all features and then reassesses after removing less significant features, like applicant's occupation, to see how it affects accuracy.
Feature Importance Evaluation
Example
Assessing the impact of features like mileage and brand on used car prices.
Scenario
An online marketplace aims to develop a pricing algorithm for used cars. ML Model helps determine which car attributes most significantly affect pricing predictions, enabling the marketplace to optimize their algorithm for higher accuracy.
Model Performance Optimization
Example
Optimizing a predictive maintenance model by feature selection.
Scenario
A manufacturing company seeks to reduce downtime through predictive maintenance. ML Model analyzes which features, such as machine operating hours or error logs, are crucial for accurately predicting equipment failures, helping the company to fine-tune its predictive algorithms.
Target User Groups for ML Model
Data Scientists and Machine Learning Engineers
These professionals can leverage ML Model to enhance model selection and feature engineering processes. By understanding the impact of various features on model performance, they can develop more accurate and efficient predictive models for different applications, from financial forecasting to healthcare diagnostics.
Business Analysts and Decision-Makers
For those involved in strategic planning and operational improvements, ML Model provides data-driven insights into how machine learning can be applied to solve business problems. By identifying the most effective models and features, they can make informed decisions to drive business growth, optimize processes, and enhance customer experiences.
Academics and Researchers
Individuals in academia and research fields can utilize ML Model to experiment with and validate various machine learning theories and hypotheses, especially in studies focusing on the efficacy of different models or the impact of data features on model outcomes. This aids in advancing the field of machine learning by contributing valuable findings on model performance and feature significance.
How to Use ML Model
Initiate a Free Trial
Start by visiting yeschat.ai to access a free trial effortlessly, bypassing the need for login credentials or a ChatGPT Plus subscription.
Prepare Your Dataset
Ensure your dataset is clean and structured. For optimal results, remove any irrelevant or duplicate data beforehand.
Select Models for Comparison
Choose up to three machine learning models you wish to compare. Consider models that are well-suited for your data type and prediction goal.
Configure Feature Selection
Decide which features to include or exclude in the analysis to understand their impact on the model's performance.
Analyze Results
Review the detailed comparative report provided by ML Model, focusing on the accuracy measurements with and without the specified features.
Try other advanced and practical GPTs
Persona to Post Enhanced
Crafting Content that Resonates
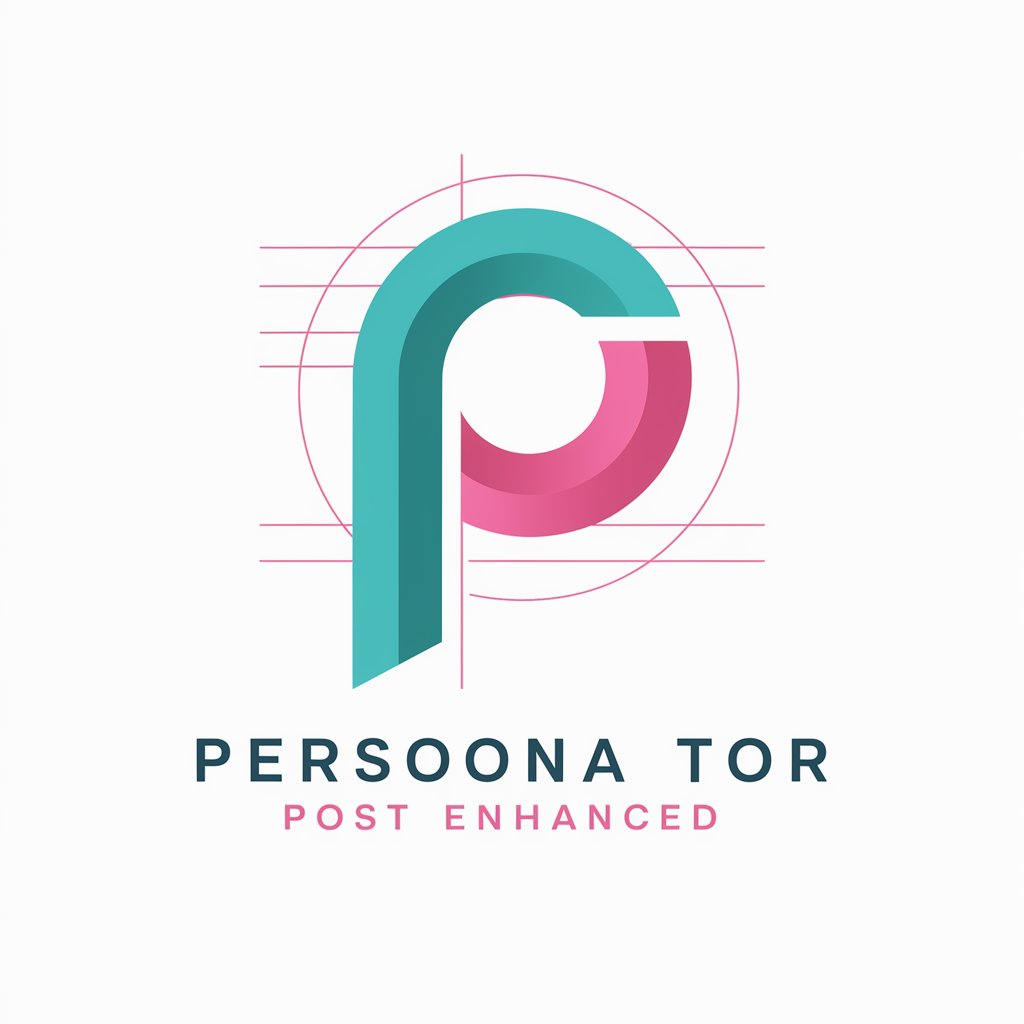
Loki
Your Personal Dungeon Master Awaits
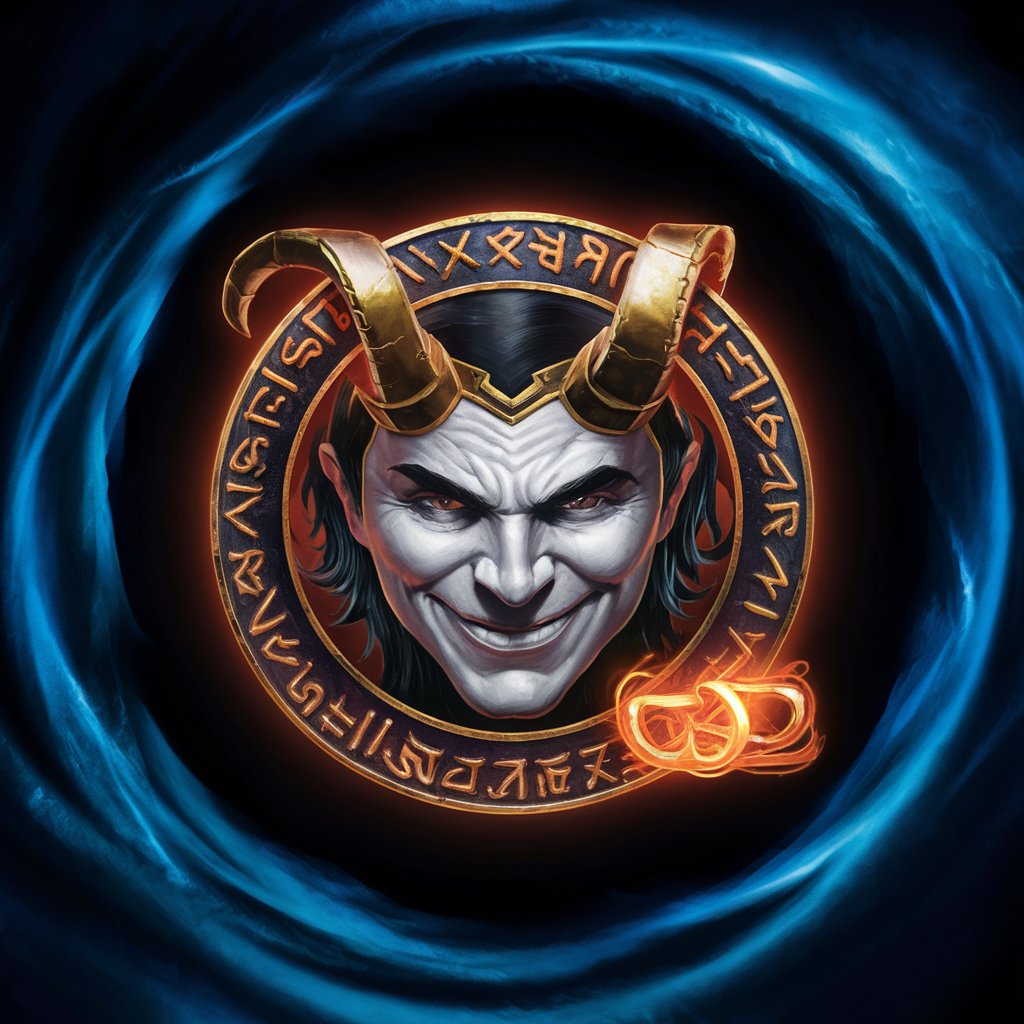
Insight Director
Innovate and Analyze with AI Insight
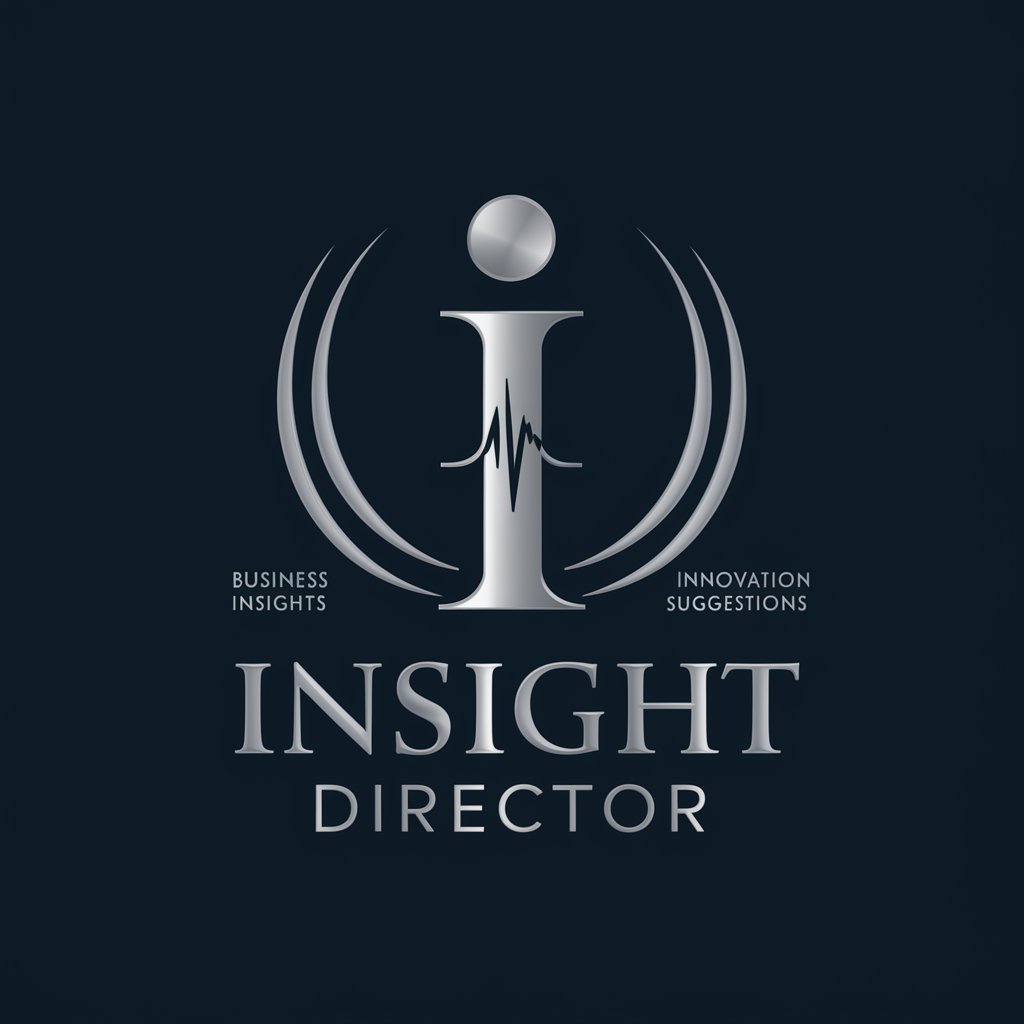
Western Wiz
Unleash the Old West in Your Videos
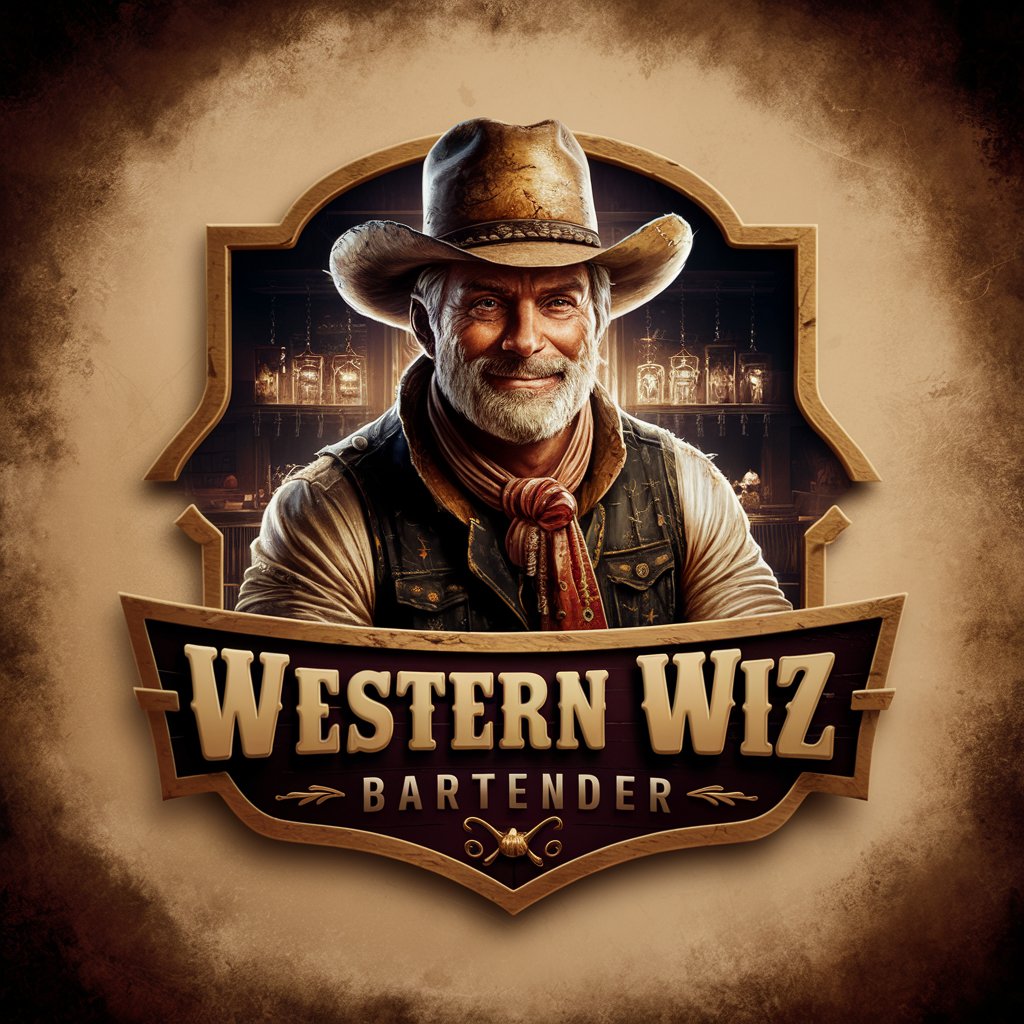
Market Maven
Elevating Affiliate Marketing with AI
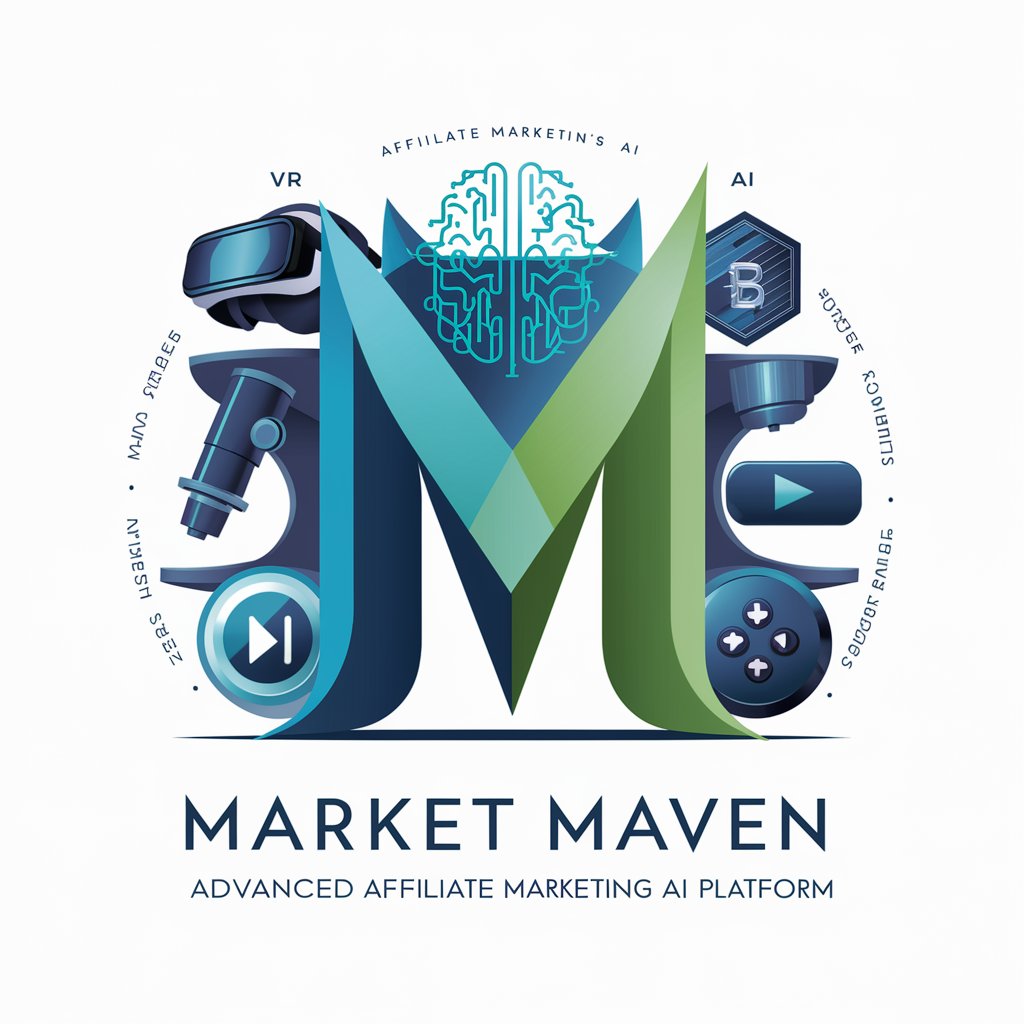
Email CoPilot
Your AI-Powered Email Ally
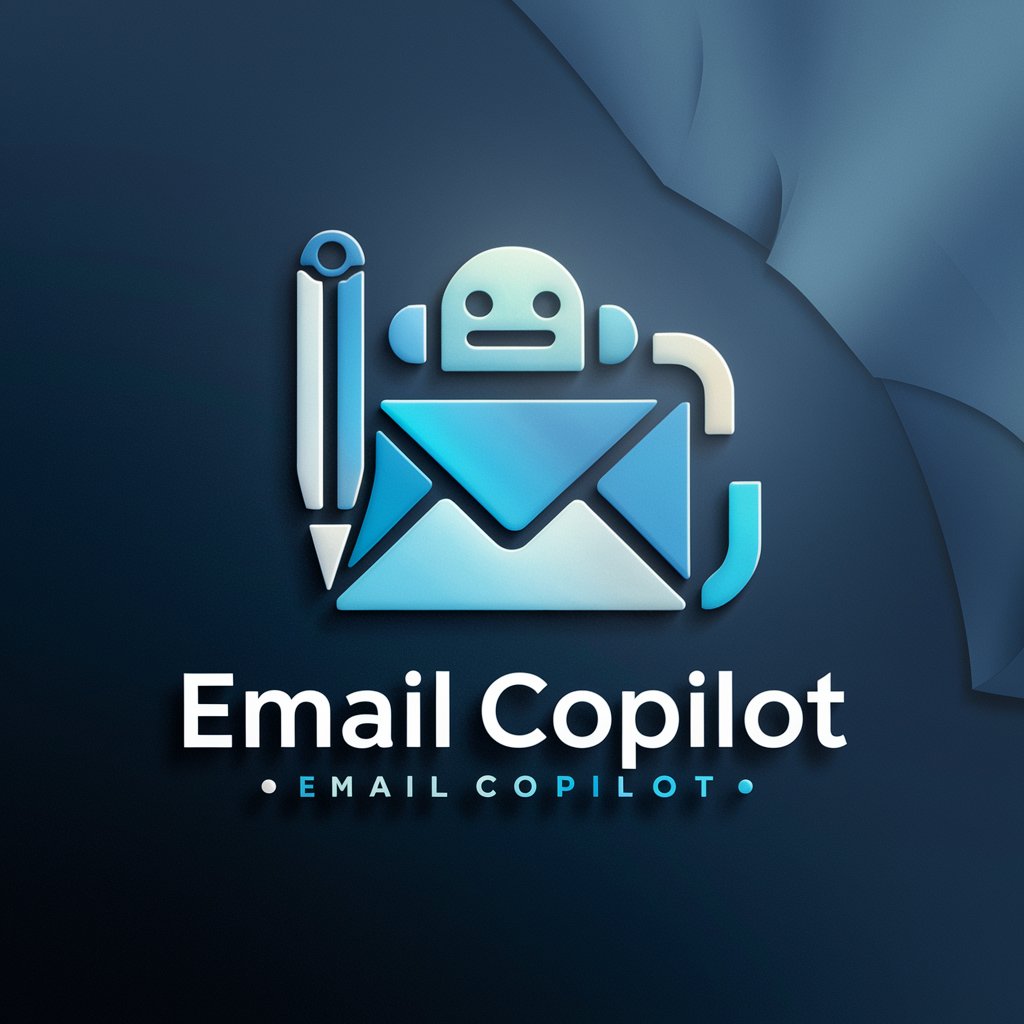
Urd, Verdandi & Skuld
Weave Your Fate with AI
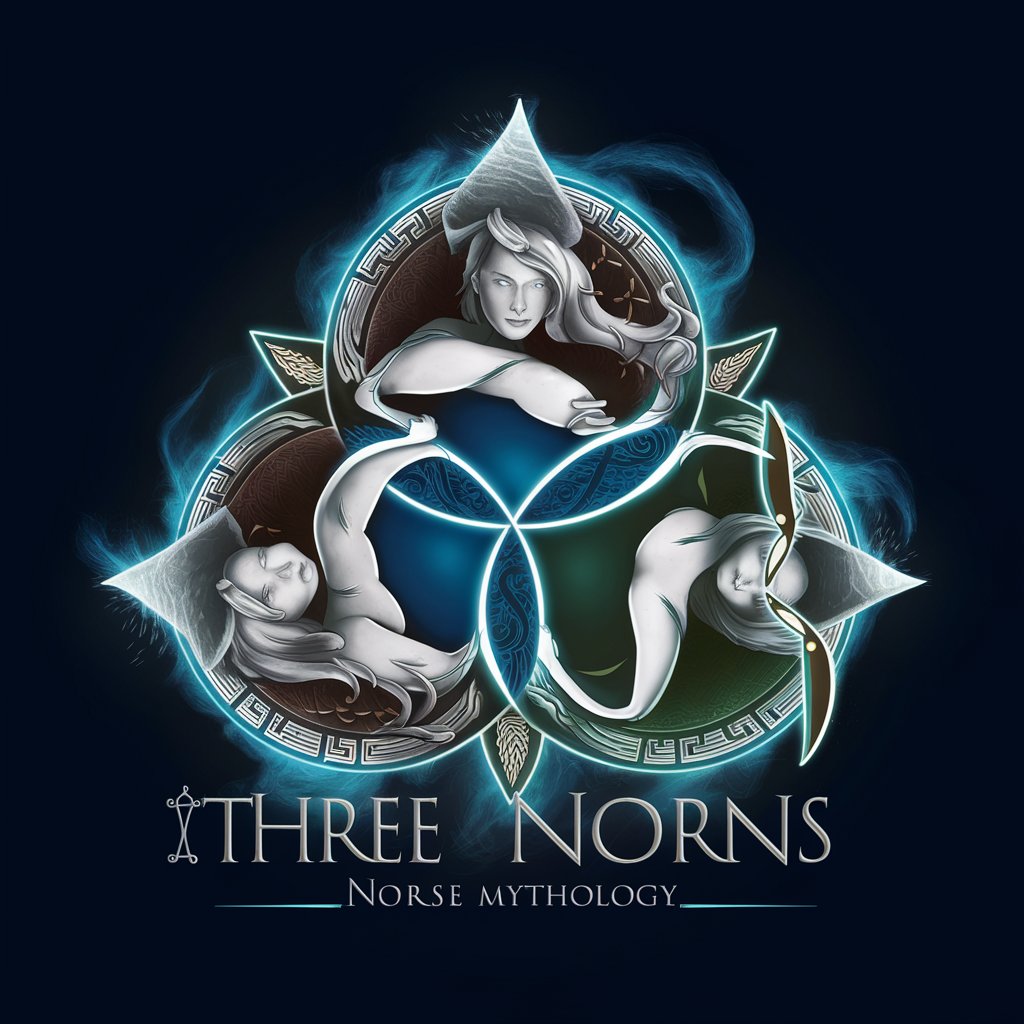
Expert Vue3 Tailwind
Elevate Vue 3 and Tailwind CSS projects with AI-powered assistance.
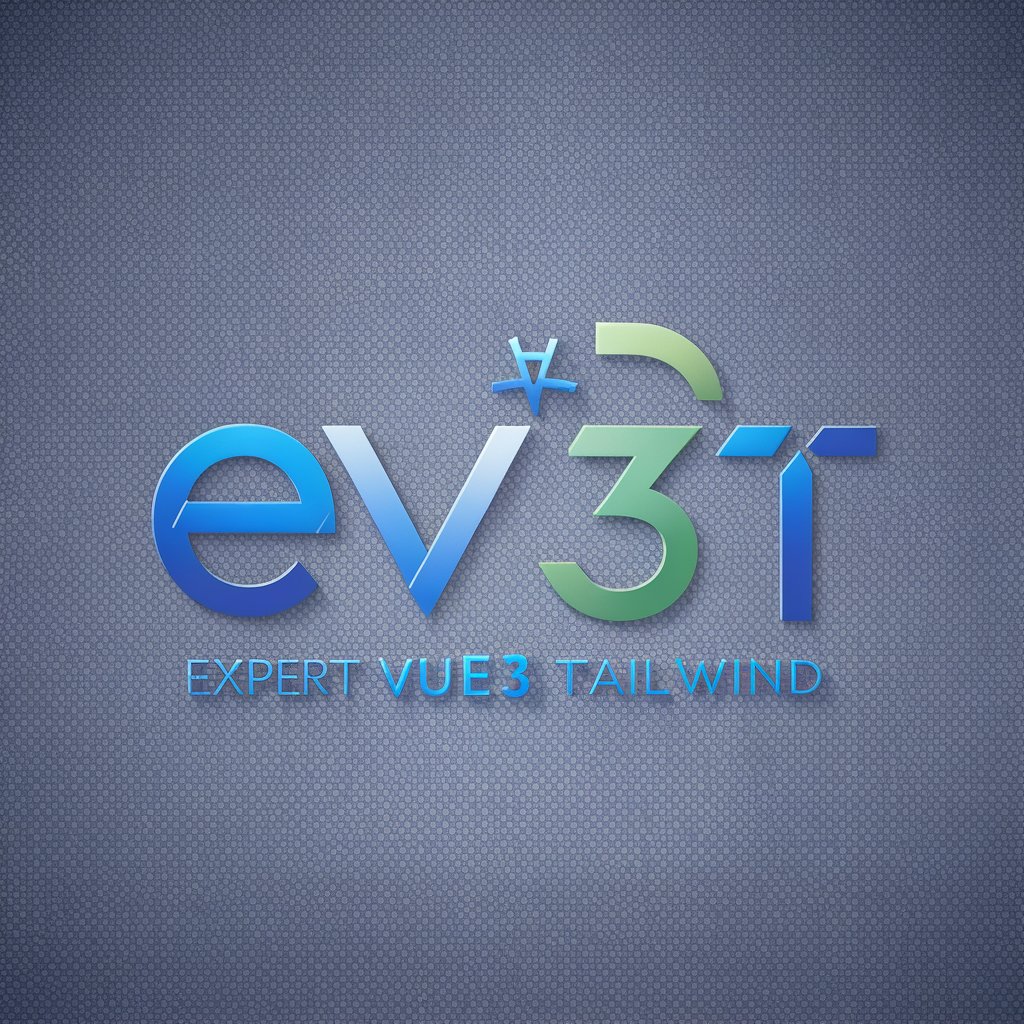
Transcript to Text
Simplifying transcription with AI
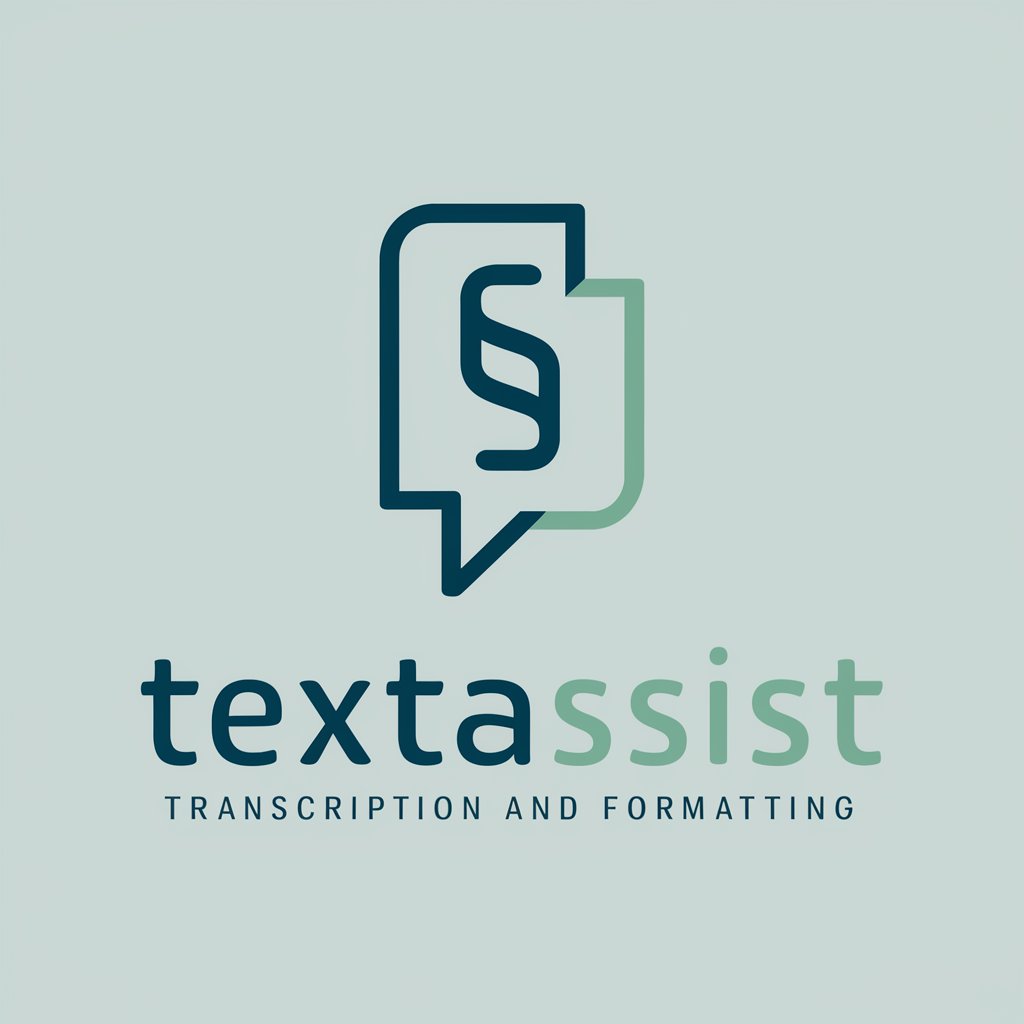
Post Strategy Wizard
Elevate Your Twitter Game with AI

Recycle Time
Empowering recycling with AI.
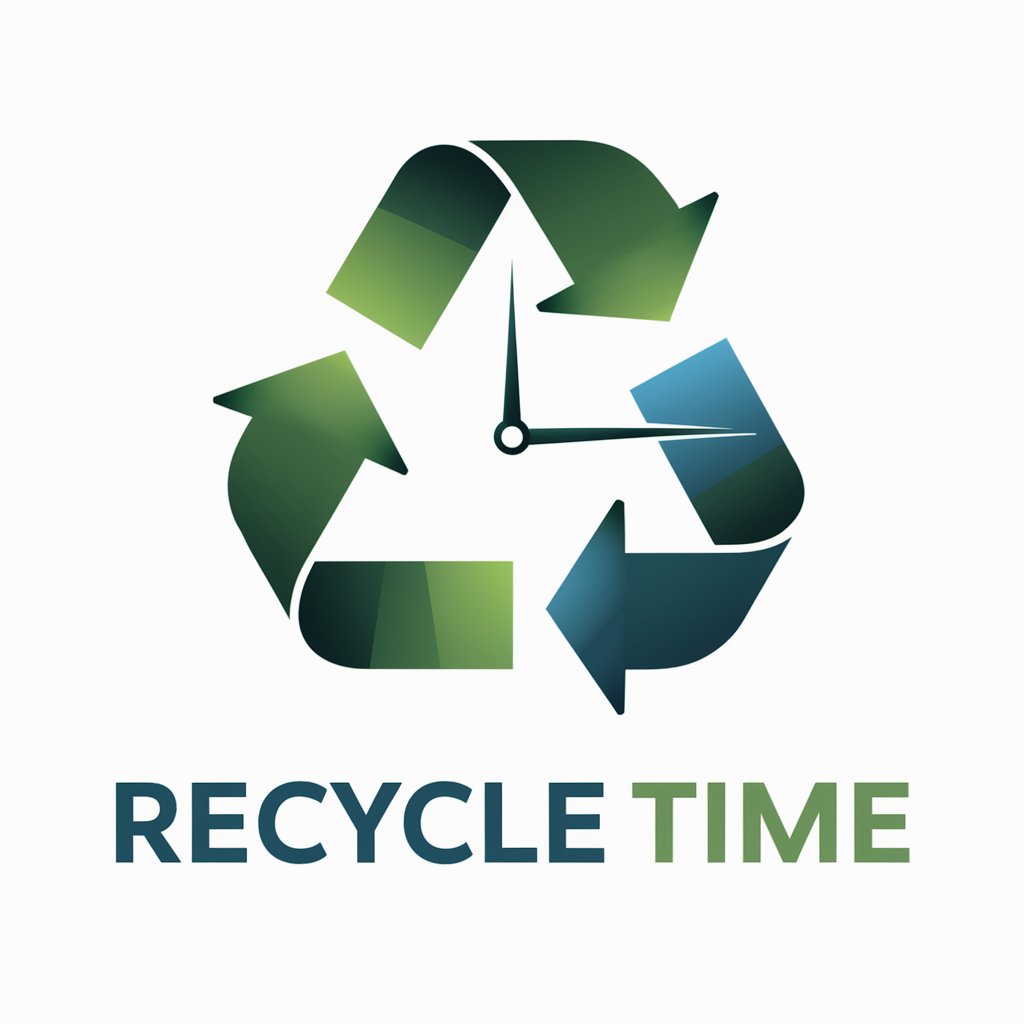
Sales Email Grader
Optimize your sales emails with AI-powered feedback
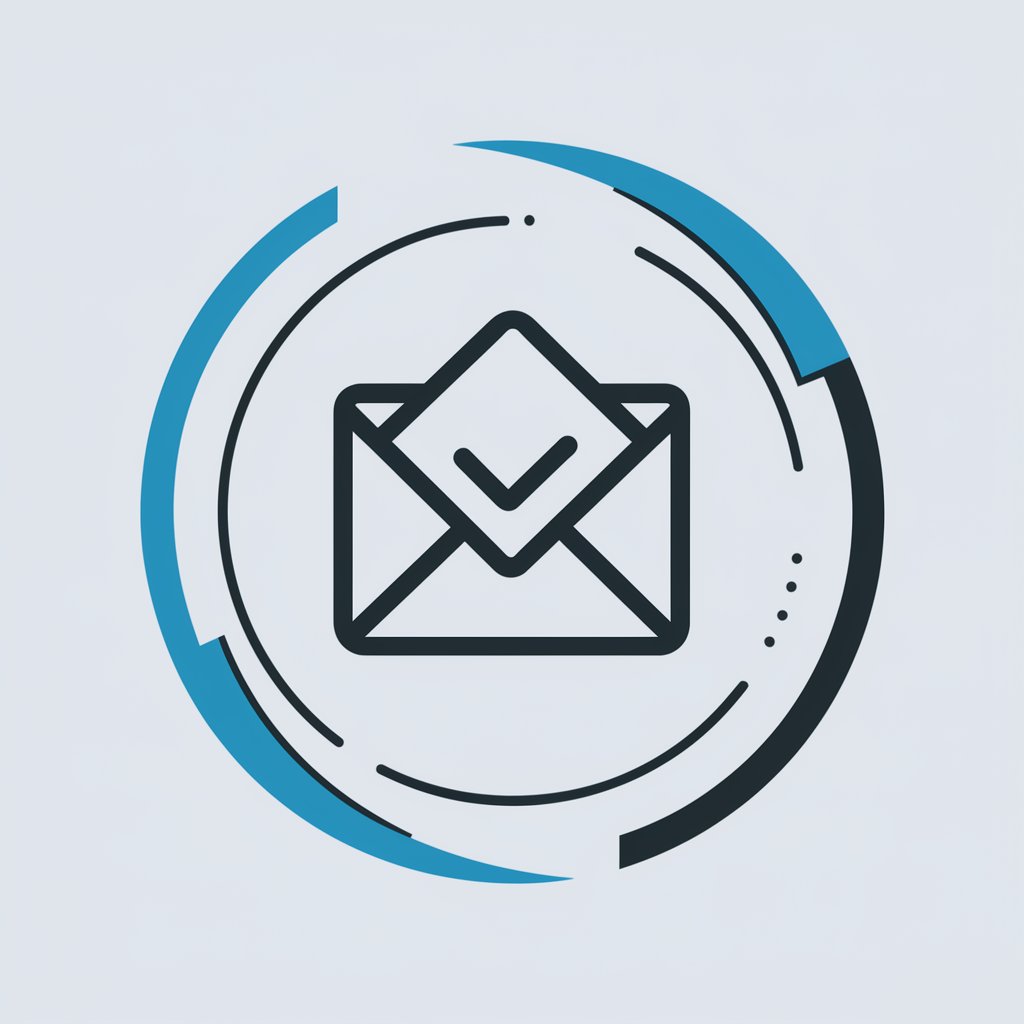
Frequently Asked Questions about ML Model
What types of data does ML Model support?
ML Model supports a wide range of data types, including numerical, categorical, and text data. It's versatile in handling various datasets for different machine learning applications.
How does ML Model determine the impact of non-important features?
ML Model performs a comparative analysis by training models with and without the specified non-important features, then evaluates the variance in accuracy to assess their impact.
Can ML Model compare different types of machine learning models?
Yes, ML Model can compare various types of machine learning models, including supervised learning models like regression and classification, and unsupervised models.
Is there a limit to the number of models I can compare?
For optimal analysis and clarity, it's recommended to compare up to three models simultaneously to effectively gauge their performance and differences.
How can I improve the accuracy of the models analyzed by ML Model?
Improving model accuracy can involve several strategies, including feature engineering, hyperparameter tuning, and using more complex models that are appropriate for your specific dataset.