Simulation Environment for Training - AI Simulation Training Platform
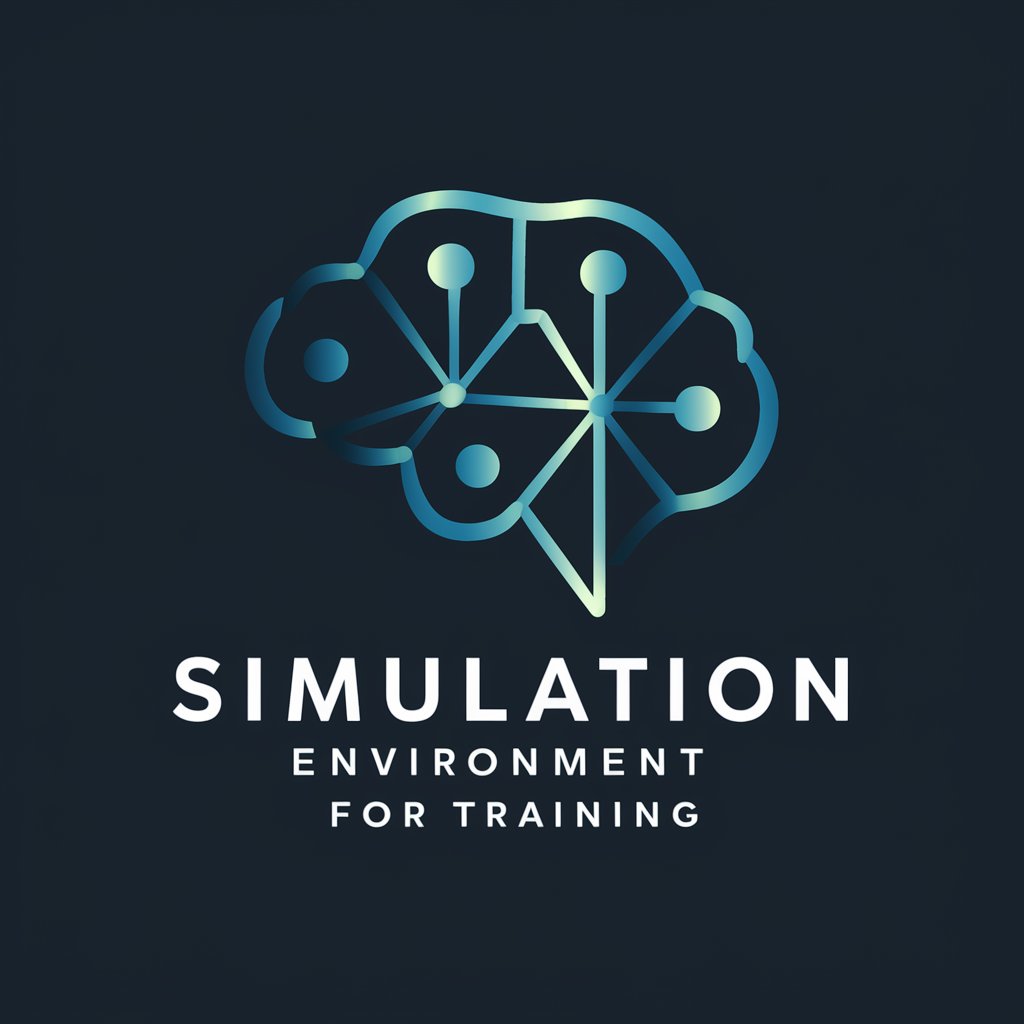
Welcome to advanced AI simulation training.
Crafting Smarter AI with Realistic Simulations
Create a detailed simulation scenario for...
How can we enhance the realism of...
What are the best practices for training AI in...
Suggest improvements for evaluating AI performance in...
Get Embed Code
Understanding Simulation Environment for Training
Simulation Environment for Training (SET) is a sophisticated platform designed to create and manage dynamic simulation environments tailored for training artificial intelligence (AI) models. By leveraging realistic, varied, and challenging scenarios, SET aims to enhance the decision-making and adaptation skills of AI systems. It focuses on providing comprehensive simulations that include dynamic environments, unpredictable variables, and intricate scenarios requiring nuanced responses. For instance, in autonomous vehicle training, SET can simulate diverse traffic conditions, weather scenarios, and pedestrian behaviors to test and improve the vehicle's decision-making algorithms under various conditions. Powered by ChatGPT-4o。
Core Functions and Applications of SET
Dynamic Scenario Generation
Example
Automatically creating diverse traffic scenarios for autonomous vehicle testing.
Scenario
SET can generate a myriad of driving conditions, from heavy rain and foggy weather to unexpected roadblocks, to train and evaluate autonomous driving systems' adaptability and decision-making in real-world conditions.
Adaptability and Response Testing
Example
Evaluating an AI healthcare assistant's responses to varied patient symptoms.
Scenario
In a healthcare simulation, SET can introduce a wide range of patient cases with different symptoms and medical histories, challenging the AI assistant to recommend appropriate care plans, thereby enhancing its diagnostic accuracy and adaptability.
Performance Evaluation Metrics
Example
Providing quantitative and qualitative metrics for AI model performance within simulations.
Scenario
SET offers a suite of analytical tools to measure AI models' effectiveness in simulations, such as precision in autonomous driving decisions or accuracy in medical diagnoses, enabling developers to fine-tune the AI systems.
Target Users of Simulation Environment for Training
AI Developers and Researchers
Professionals in AI development and research who require sophisticated environments to train, test, and refine their AI models. They benefit from SET's capability to simulate complex, real-world scenarios that are crucial for developing robust and adaptable AI systems.
Educational Institutions
Academic institutions offering courses in AI, robotics, and other related fields can utilize SET to provide students with hands-on experience in training AI models, enabling a practical understanding of AI challenges and solutions.
Corporate Training Departments
Corporate training teams can employ SET to simulate real-world business scenarios for training AI systems in areas such as customer service, predictive maintenance, or operational optimization, enhancing their effectiveness and efficiency.
Guidelines for Using the Simulation Environment for Training
Begin Your Journey
Start by exploring yeschat.ai for an introductory trial, accessible without any login requirements or the need for a ChatGPT Plus subscription.
Identify Your Training Needs
Determine the specific AI training objectives you aim to achieve, such as improving decision-making, enhancing predictive accuracy, or simulating complex scenarios.
Customize Your Simulation
Utilize the platform's tools to tailor your simulation environment. This can include setting variables, defining parameters, and selecting scenarios that closely mimic your real-world applications.
Engage in the Simulation
Run your AI models within the created simulation, observing their decisions, reactions, and adaptations to the dynamic environment and challenges presented.
Analyze and Iterate
Leverage the platform's analytical tools to evaluate your AI model's performance. Use these insights to make iterative improvements to both your model and the simulation environment for optimal outcomes.
Try other advanced and practical GPTs
Miracle Guide
Empowering your journey with divine guidance
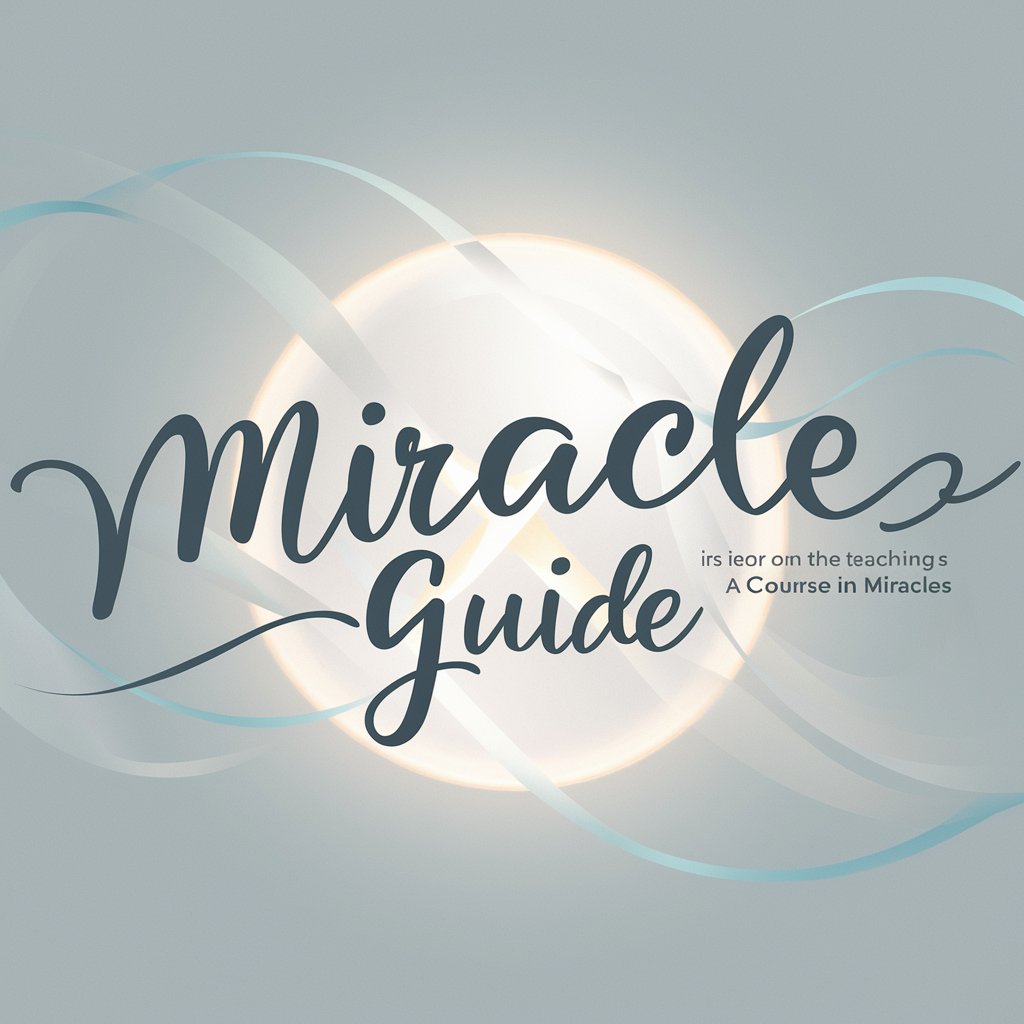
Miru [Miracle Story Teller]
Crafting your narratives, powered by AI
![Miru [Miracle Story Teller]](https://r2.erweima.ai/i/6dowaqEdSwW0j-zmmERD3g.png)
Miracle Sports Predictor (기적의 스포츠 승부 예측)
Predicting sports outcomes with AI-powered precision
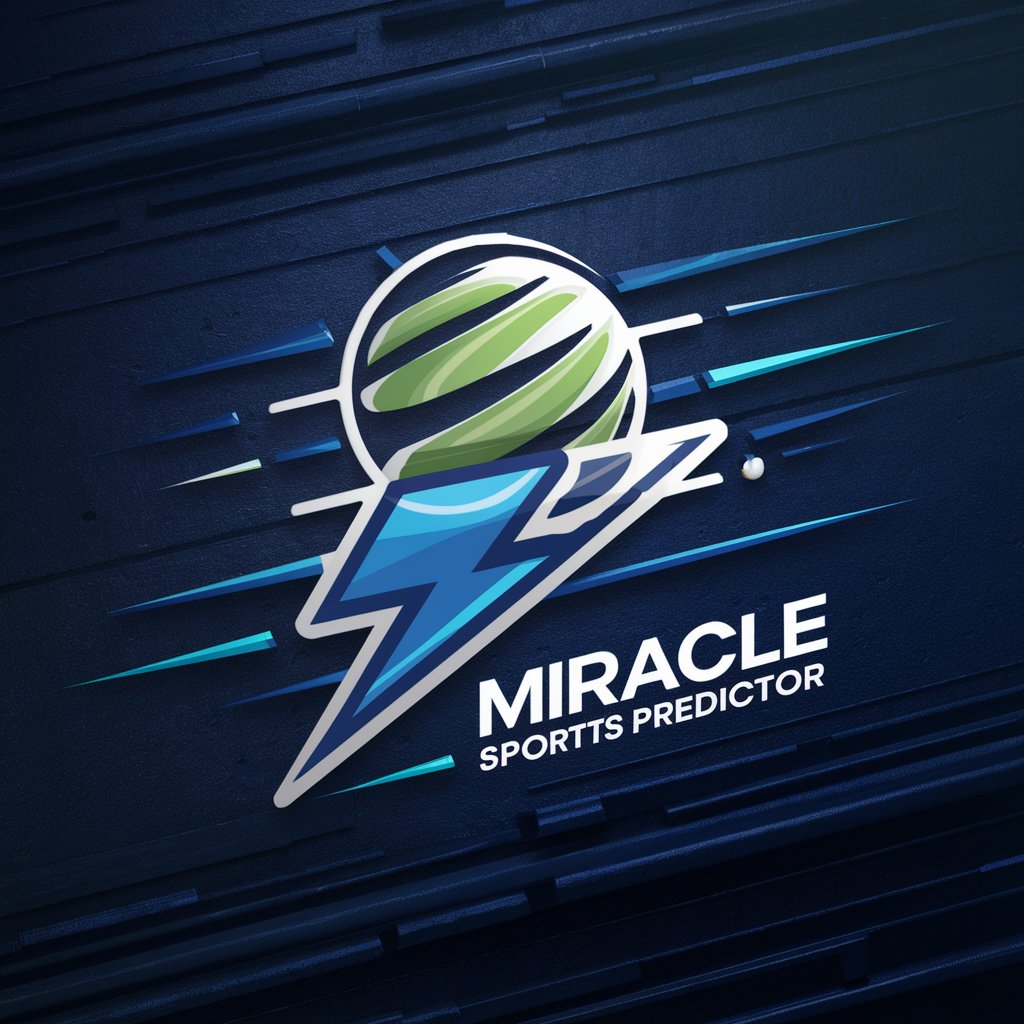
Ukrainian Economic Miracle
Revolutionizing Economics with AI
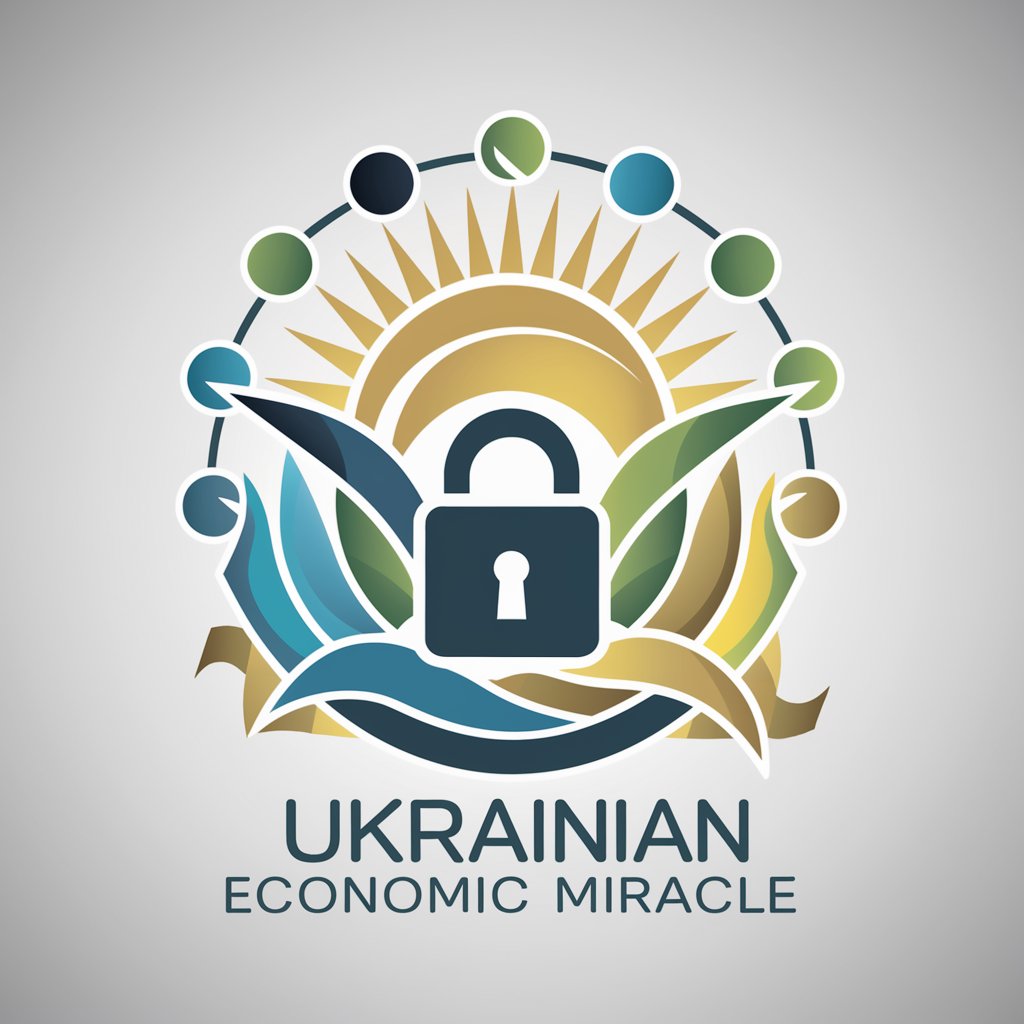
Mirakle Letter GPT
Crafting respectful letters with AI
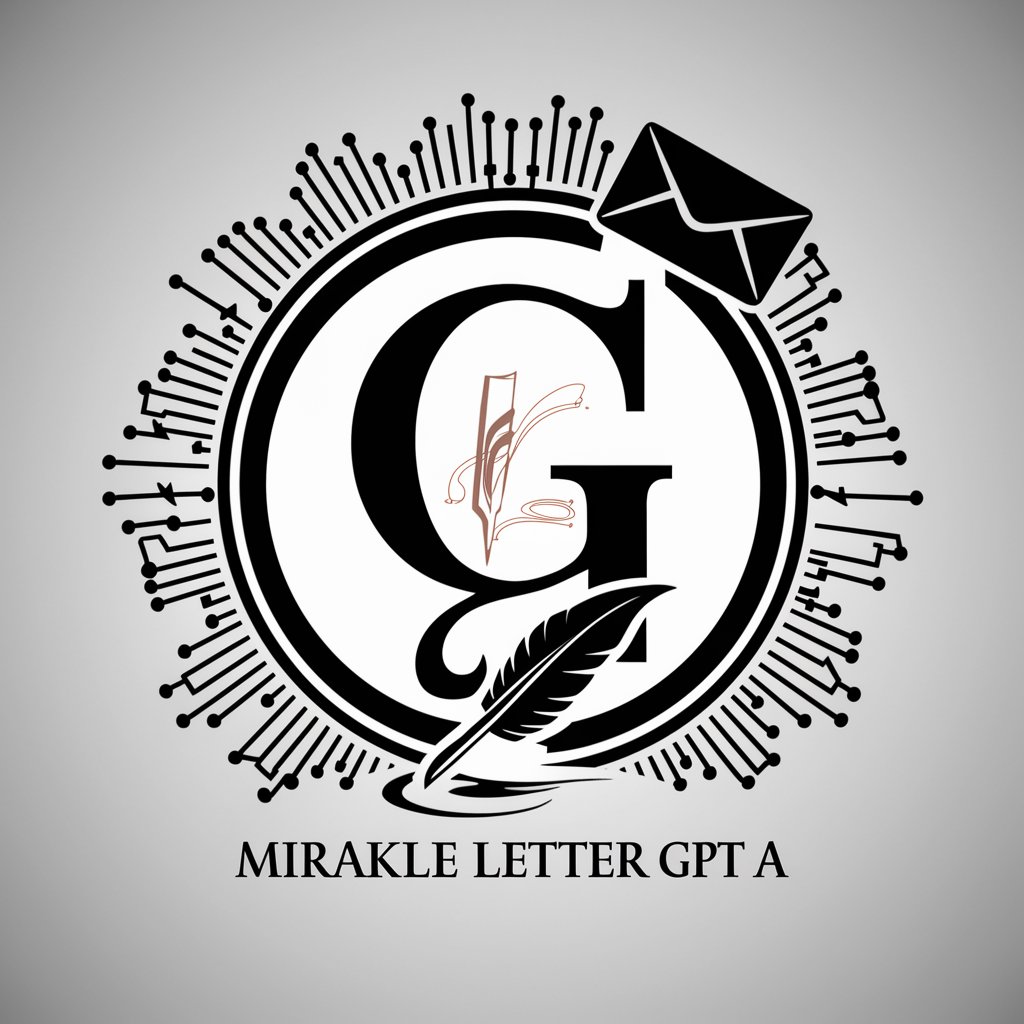
Miracle Guide
Transforming spirituality with AI insight.
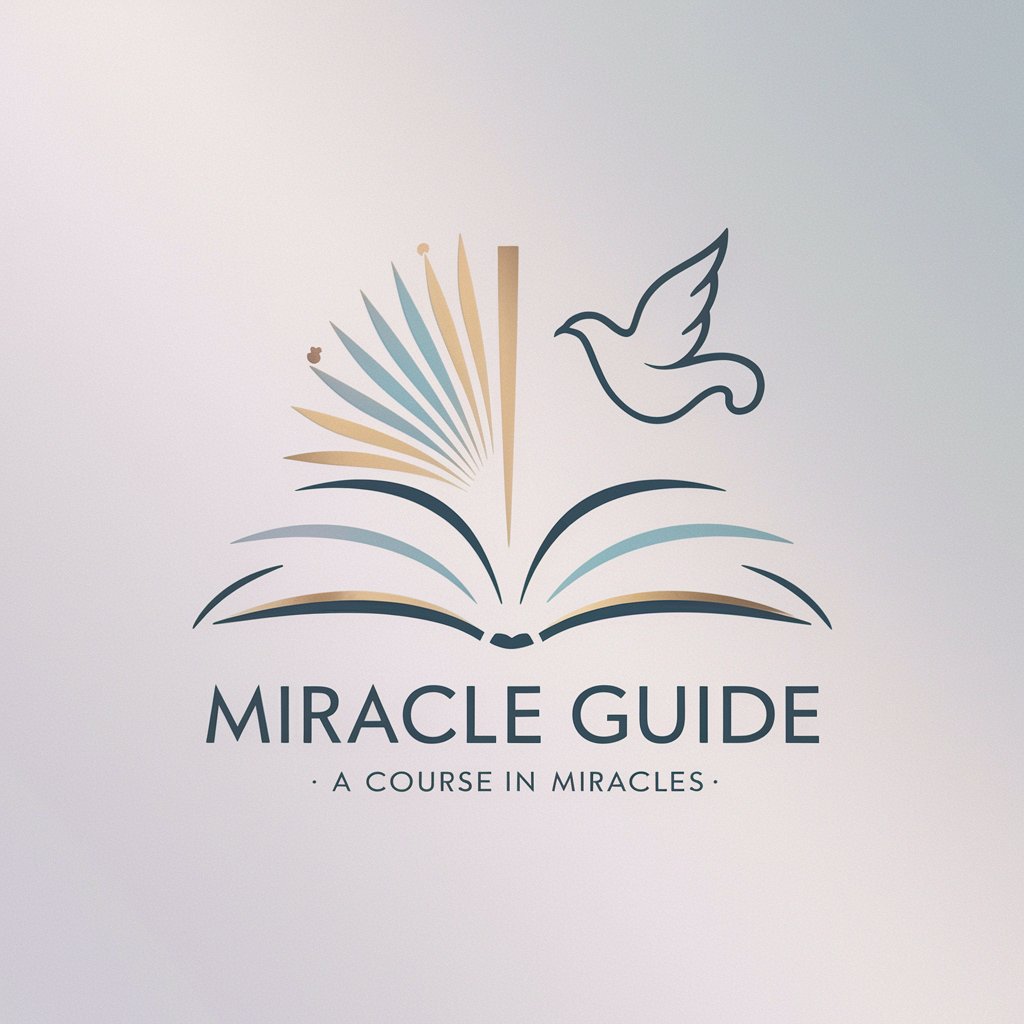
Matrix of Environment Creation
Craft worlds with AI-powered imagination
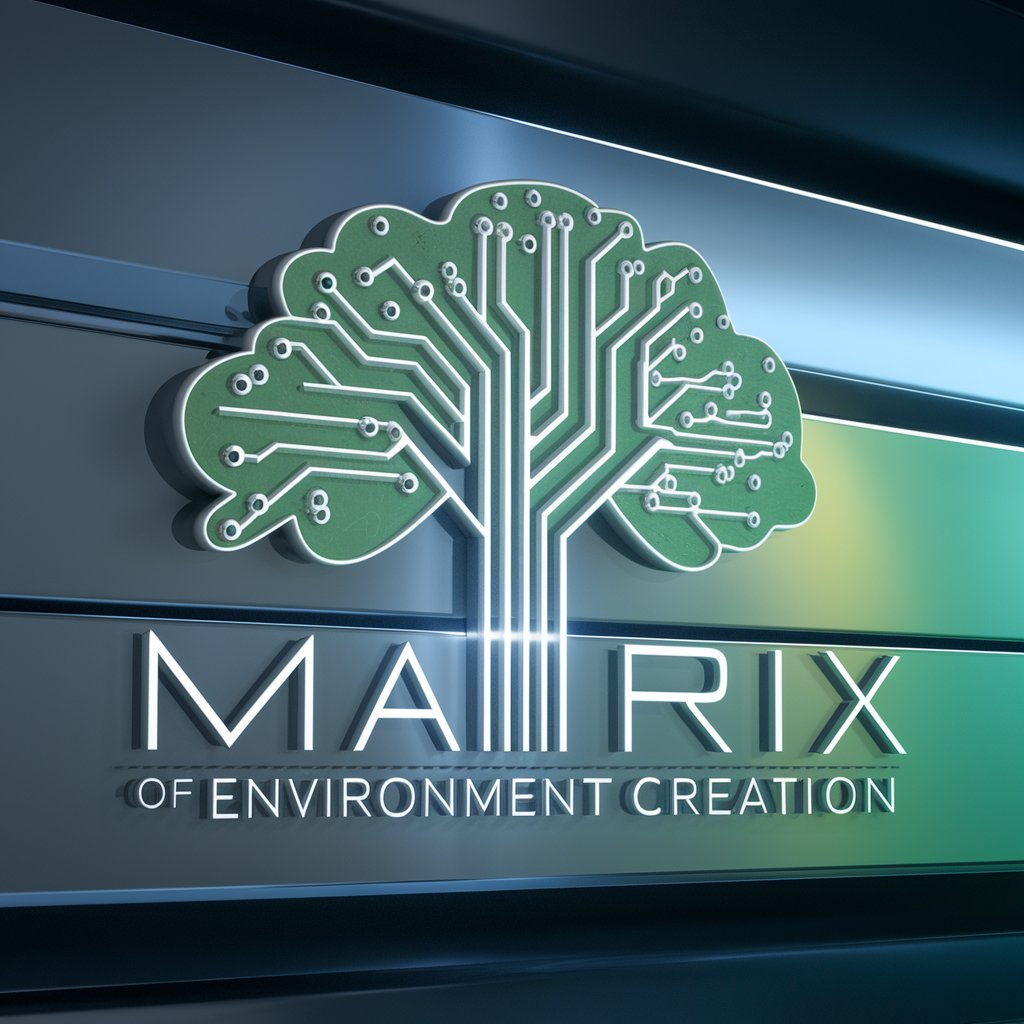
Health & Environment Analyst
Transforming Data into Environmental Action
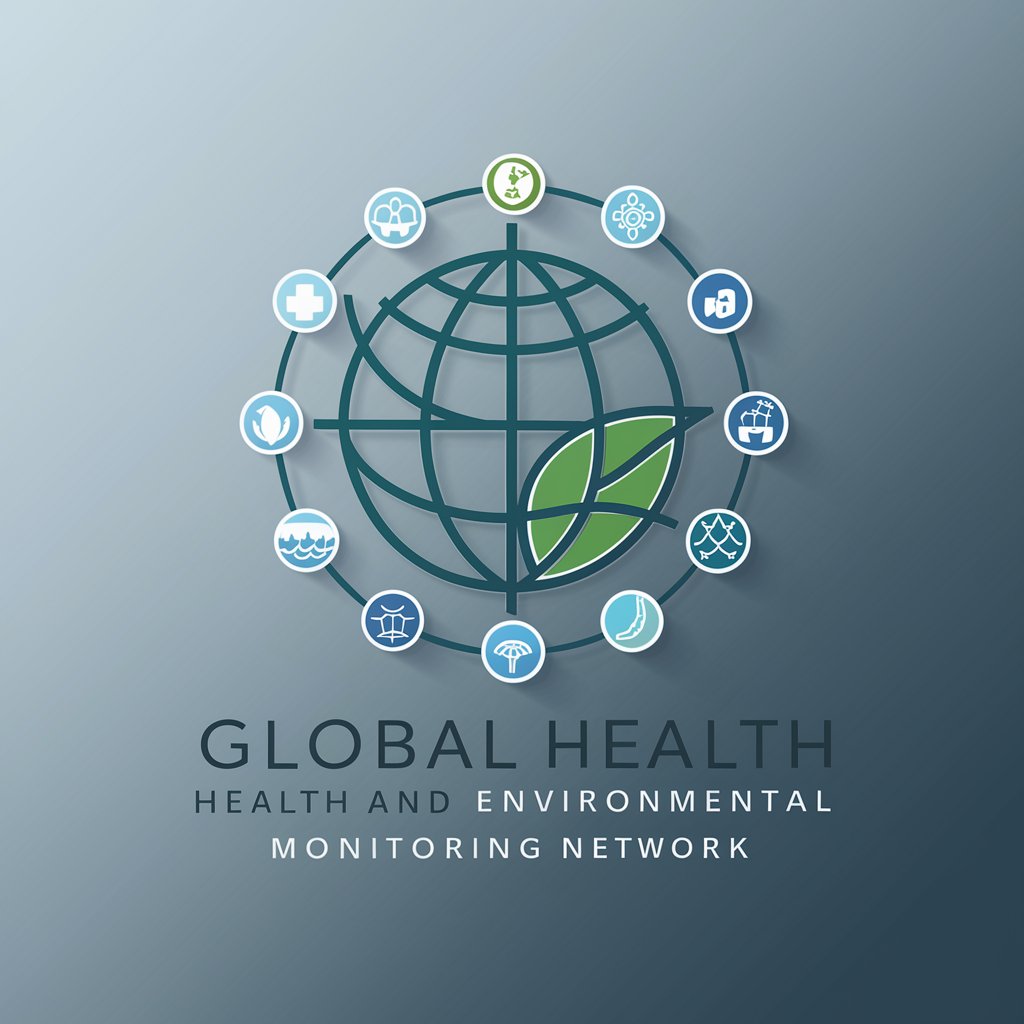
Cybersecurity Simulation Environment
AI-driven Cybersecurity Training and Simulation
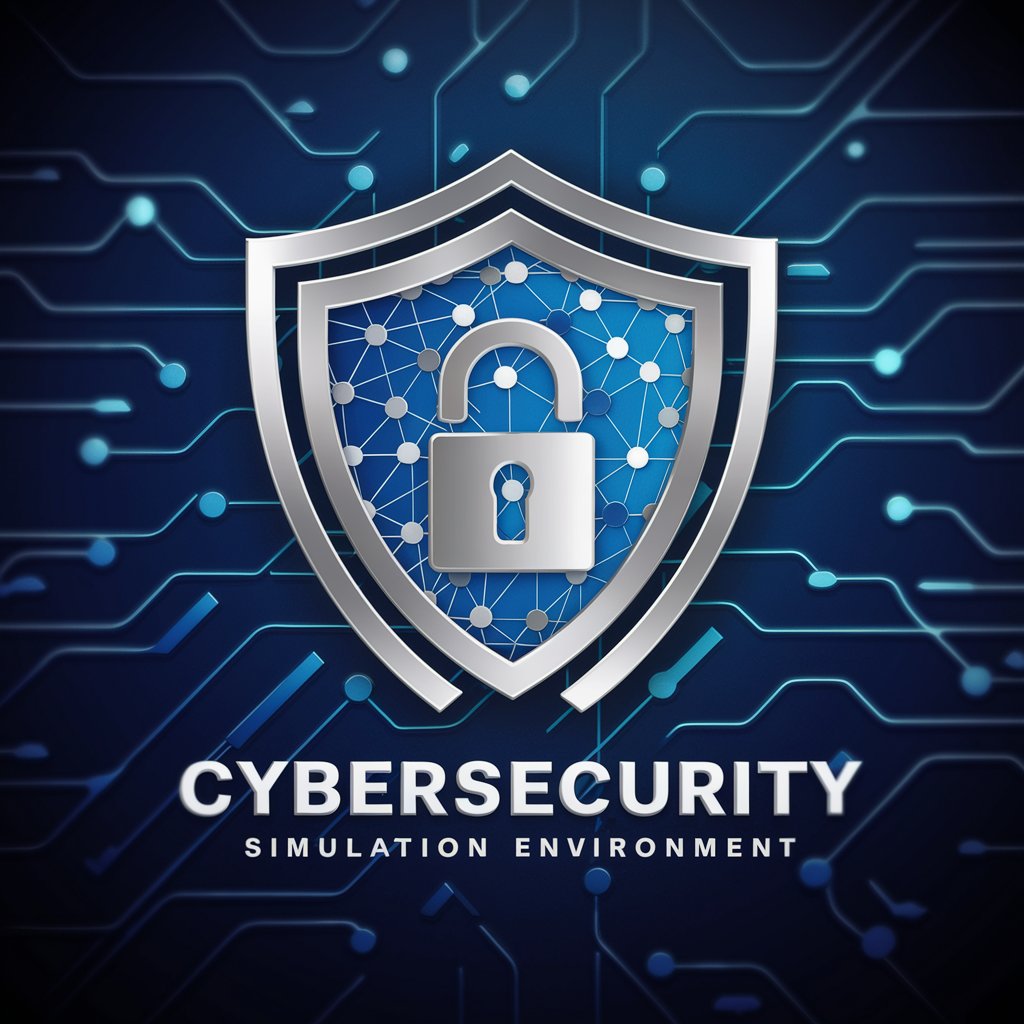
Environment Professor GPT
Empowering Environmental Learning with AI

Docker environment creator
Streamline Docker setup with AI
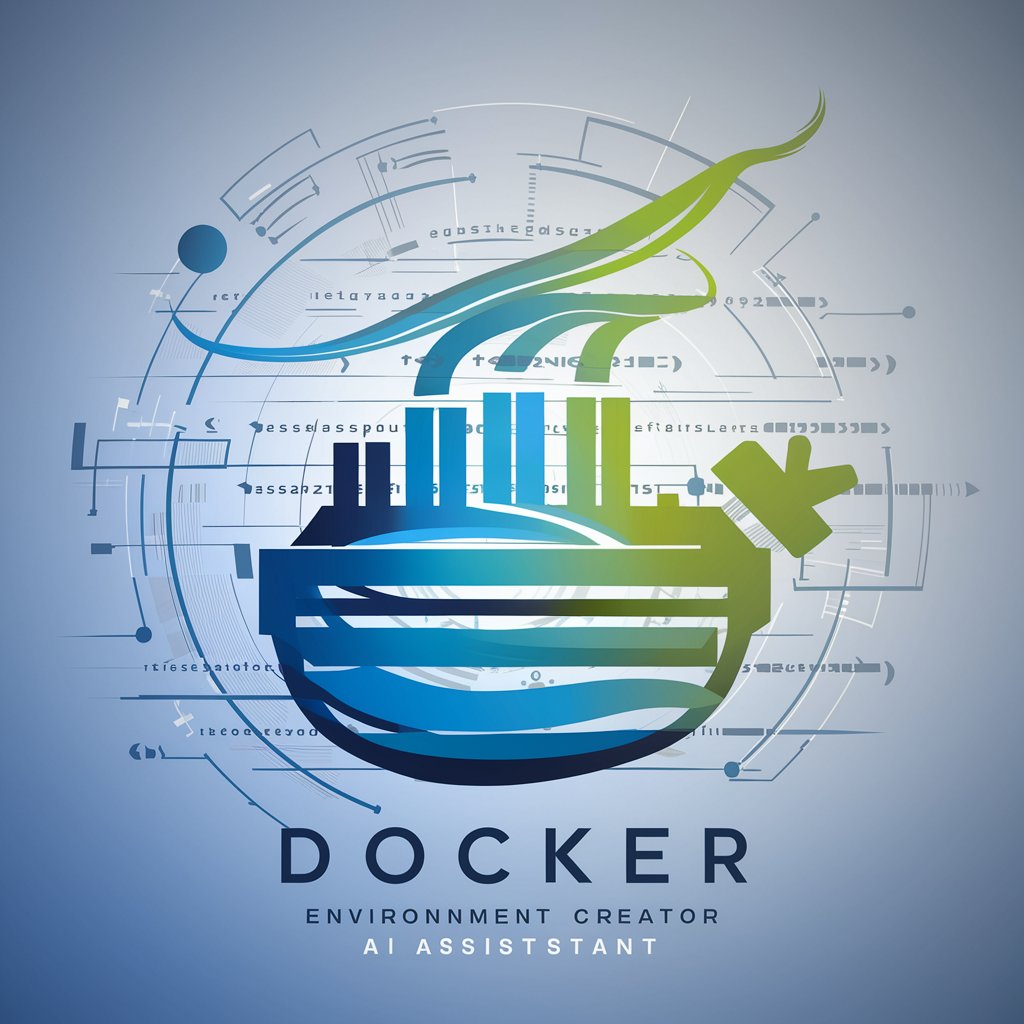
Pro Coding Environment
Elevate Your Coding Skills with AI-Powered Assistance
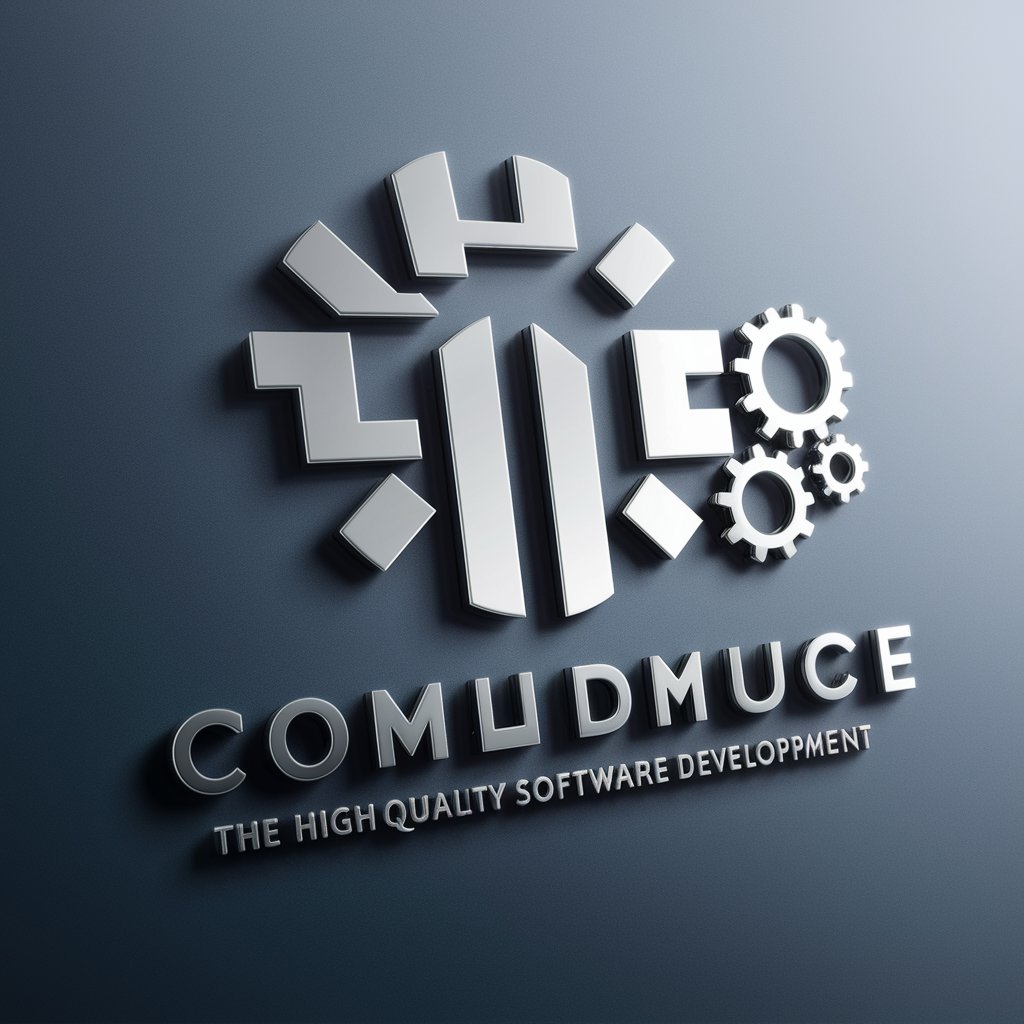
Frequently Asked Questions about the Simulation Environment for Training
What makes the Simulation Environment for Training unique?
This platform offers a highly customizable and dynamic simulation environment designed to train AI models in complex scenarios. Its unique feature lies in the ability to create varied and challenging situations that require nuanced responses, enabling the development of AI with advanced decision-making capabilities.
Can I use this platform for any type of AI model?
Yes, the Simulation Environment for Training is versatile and supports a wide range of AI models. Whether you're working on natural language processing, computer vision, or decision-making algorithms, the platform can accommodate your training needs.
How can I measure the performance of my AI within the simulations?
The platform provides comprehensive analytical tools that allow you to track various performance metrics of your AI models during the simulations. These metrics can include accuracy, decision-making speed, and adaptability to changing scenarios.
Is there support for beginners who are new to AI training?
Absolutely. The platform offers extensive documentation, tutorials, and customer support to guide users through the setup and customization of their simulation environments, making it accessible for beginners and advanced users alike.
Can the simulation environments be scaled to represent real-world complexities?
Yes, one of the platform’s strengths is its scalability. You can adjust the complexity and scale of the simulation environments to closely replicate real-world conditions, ensuring your AI models are well-prepared for actual applications.